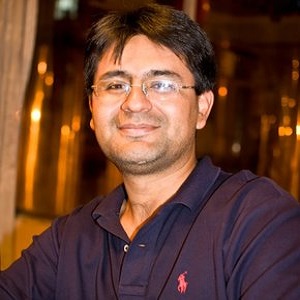
Mauricio Villarroel
Senior Researcher Digital Health, Co-Lead CameraLab, Institute of Biomedical Engineering, Department of Engineering Science, University of Oxford
Title of the talk
“Physiological monitoring of preterm infants in the Neonatal Intensive Care Unit”
The implementation of video-based non-contact technologies to monitor the vital signs of preterm infants in the hospital presents several challenges, such as the detection of the presence or the absence of a patient in the video frame, robustness to changes in lighting conditions, automated identification of suitable time periods and regions of interest from which vital signs can be estimated. We carried out a clinical study to evaluate the accuracy and the proportion of time that heart rate and respiratory rate can be estimated from preterm infants using only a video camera in a clinical environment, without interfering with regular patient care. A total of 426.6 h of video and reference vital signs were recorded for 90 sessions from 30 preterm infants in the Neonatal Intensive Care Unit (NICU) of the John Radcliffe Hospital in Oxford. Each preterm infant was recorded under regular ambient light during daytime for up to four consecutive days. We developed multi-task deep learning algorithms to automatically segment skin areas and to estimate vital signs only when the infant was present in the field of view of the video camera and no clinical interventions were undertaken. We propose signal quality assessment algorithms for both heart rate and respiratory rate to discriminate between clinically acceptable and noisy signals. The mean absolute error between the reference and camera-derived heart rates was 2.3 beats/min for over 76% of the time for which the reference and camera data were valid. The mean absolute error between the reference and camera-derived respiratory rate was 3.5 breaths/min for over 82% of the time. Accurate estimates of heart rate and respiratory rate could be derived for at least 90% of the time, if gaps of up to 30 seconds with no estimates were allowed.
Speaker Bio
Mauro completed his undergraduate engineering degrees in South America and his doctorate degree in Engineering Science from the University of Oxford. As a research scientist at the Massachusetts Institute of Technology, he collaborated for several years with major hospitals and companies to develop a number of advanced monitoring algorithms and concepts to improve the efficiency, accuracy and timeliness of clinical decision making in intensive care. He was instrumental in the development and dissemination of the Intelligent Monitoring in Intensive Care (MIMIC) II database, one of the first public research resources containing detailed medical records from tens of thousands of patients from several Intensive Care Units. Since 2010, as a senior researcher at the Institute of Biomedical Engineering in the University of Oxford, Mauro leads a research group for the development of probabilistic models and machine learning algorithms using video cameras and wearable sensors to understand the underlying physiological state of both adult patients and premature infants, especially those with moderate to severe long-term conditions. His major focus is in video and image analysis for the non-intrusive/non-contact physiological measurement of patients’ vital signs, activity and an understanding of the visual environment in a hospital setting.